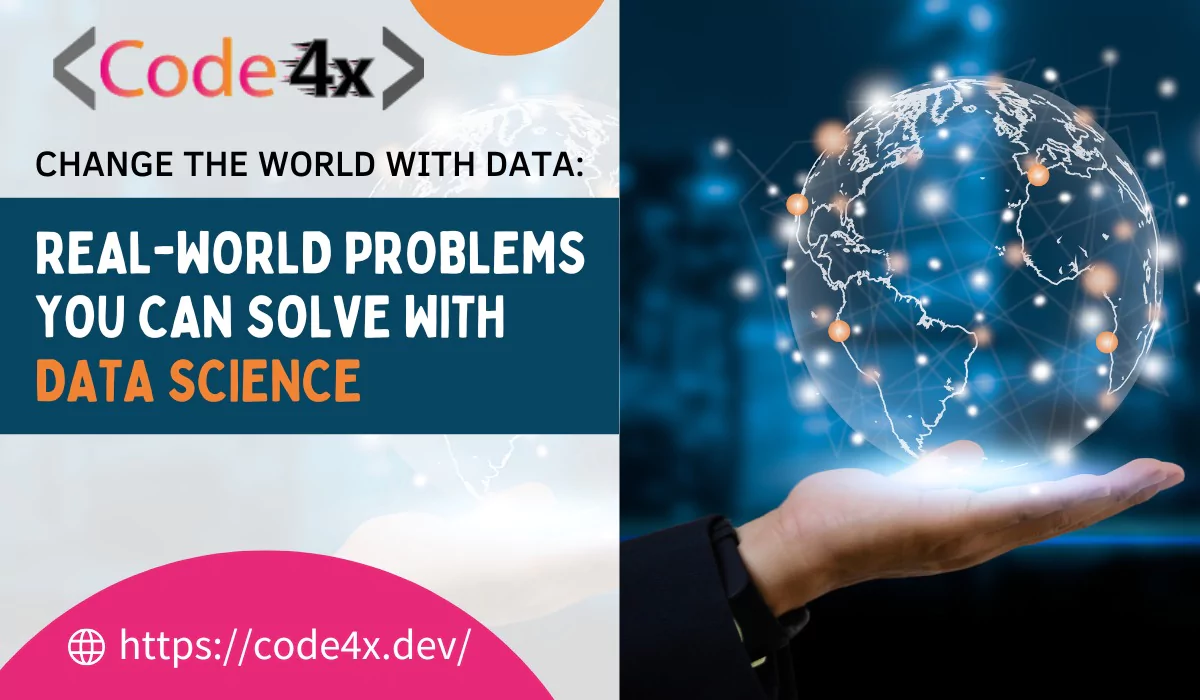
Change the World with Data – Real-World Problems You Can Solve with Data Science
Data science isn’t just about fancy algorithms and complex equations. It’s about using the power of information to tackle real-world challenges that impact our lives every day. Feeling intrigued but unsure where to start? This blog is for you! Let’s dive into some inspiring examples of how data science is making a difference, and how YOU can be part of it, along with the specific techniques and skills you can learn to make it happen –
1. Healthcare Hero –
Ever dreamt of saving lives through data? Data science is revolutionizing healthcare by –
a. Predicting and preventing diseases –
- Techniques – Machine learning algorithms like random forests and support vector machines can analyze medical records to identify patterns and risk factors for diseases like heart disease, diabetes, or cancer.
- What to learn – Statistics, machine learning, data visualization, and an understanding of medical terminology.
b. Developing personalized medicine –
- Techniques – Natural Language Processing (NLP) can analyze genetic data and medical history to suggest personalized treatment plans, while clustering algorithms can group patients with similar characteristics for targeted therapies.
- What to learn – NLP, bioinformatics, statistics, and familiarity with clinical research methods.
c. Unveiling drug secrets
- Techniques – Deep learning models can analyze large libraries of molecules to identify potential drug candidates, while reinforcement learning can optimize their development process.
- What to learn – Deep learning, computational biology, and an understanding of drug discovery pipelines.
2. Finance whiz –
Want to make a positive impact on the financial world? Data science can help –
a. Fight fraudsters –
- Techniques – Anomaly detection algorithms can flag suspicious transactions in real-time, while graph theory can identify networks of fraudulent activity.
- What to learn – Machine learning, anomaly detection, and familiarity with financial transaction data.
b. Predict market trends –
- Techniques – Time series analysis and forecasting models can predict market movements based on historical data and market sentiment analysis.
- What to learn – Statistics, time series analysis, and financial modeling.
c. Democratize finance –
- Techniques – Recommendation systems can suggest personalized financial products based on individual needs and goals, while natural language processing can analyze investor sentiment to gauge market trends.
- What to learn – Recommendation systems, NLP, and an understanding of financial products and regulations.
3. Retail Rockstar –
Ever wondered how online stores recommend products you actually want? Data science is the magic behind it –
a. Product recommendations –
- Techniques – Collaborative filtering algorithms recommend products based on similar users’ purchase history, while content-based filtering suggests items based on product attributes and user preferences.
- What to learn – Recommendation systems, data mining, and an understanding of customer behavior.
b. Pricing perfection –
- Techniques – A/B testing and dynamic pricing algorithms can optimize prices based on customer demand and market conditions.
- What to learn – Statistics, A/B testing, and pricing strategies.
c. Supply chain smarts –
- Techniques – Network optimization algorithms can optimize delivery routes and inventory levels, while forecasting models can predict demand fluctuations.
- What to learn – Operations research, logistics, and supply chain management principles.
4. Environmental Champion –
Concerned about our planet? Data science is on the frontlines of –
a. Combating climate change –
- Techniques – Climate models can predict future climate scenarios based on historical data and human activity, while remote sensing can monitor changes in environmental indicators.
- What to learn – Climate science, remote sensing, and statistical modeling.
b. Monitoring pollution –
- Techniques – Sensor data analysis can track pollution levels in real-time, while geospatial analysis can identify sources of pollution.
- What to learn – Data visualization, geospatial analysis, and environmental science fundamentals.
c. Conserving resources –
- Techniques – Energy demand forecasting models can optimize energy usage, while smart grid technologies can integrate renewable energy sources.
- What to learn – Energy analytics, sustainability principles, and familiarity with smart grid technologies.
Remember, the power to solve real-world problems lies within you. By focusing on specific Data Science techniques and skills relevant to your area of interest, you can equip yourself to make a meaningful impact on the world. Are you ready to unlock it?